For more stories like this, sign up for the PLANADVISERdash daily newsletter.
Using Data to Determine the Best QDIA for Your Clients
A paper from Morningstar Investment Management lays out a framework for advisers and plan sponsors to determine the qualified default investment alternative (QDIA) that is the best fit for a plan's demographics.
Recent technological advancements, along with increased data availability and quality, enable plan sponsors to stop guessing and move beyond heuristics to use detailed data on individual participants to make a robust, data-driven selection of an appropriate qualified default investment alternative (QDIA). Morningstar Investment Management staff detail this in their new paper, “Stop Guessing: Using Participant Data to Select the Optimal QDIA.”
Thomas Idzorek, CFA, chief investment officer – Retirement at Morningstar Investment Management LLC in Chicago, and lead author of the paper, tells PLANADVISER, “Our managed account engine will consider age, plan account balance, salary, contribution, state of residence—different states have different tax rates—employer tiered match, employer contribution, plan loans, brokerage account holdings, retirement age, gender and pension as well as other outside assets to determine the recommended allocation to equities for each participant.” Much of this information the plan recordkeeper would have, he notes.
The paper shows how knowing this information can help defined contribution (DC) plan sponsors determine the QDIA that best fits their participant base.
To demonstrate the framework, Idzorek explains, the team first ran a hypothetical plan of 10 participants of different ages through Morningstar’s managed account engine to come up with a recommended allocation to equities. They first plotted each individual’s recommended allocation to equities on a chart, alongside the equity glide path for a balanced fund. When measuring the difference in equities between the glide path and the individual recommendations, they found the balanced fund was off by an average of 20 equity percentage points. They then plotted each individual’s recommended equity allocation alongside the equity glide path of the Morningstar Lifetime Allocation Index – Conservative and found it was off by only 12 equity percentage points.
“If both cost the same, the plan sponsor should choose the one that fits its demographic better,” Idzorek says. He notes that this same comparison can be done with funds offered by any provider or any QDIA a plan sponsor wants to evaluate.
The report authors did the same comparison to the Morningstar Lifetime Allocation Index – Moderate, as well as a custom glide path. “A custom glide path may cost more, but it could improve fit even more,” Idzorek points out. He adds that for some plans that have participants with diverse financial situations, the recommended equity allocation “may be all over the place, and in that case, a managed account may be the best fit.”
Where does cost come into the decision? If the plan sponsor could improve the average fit for its demographic, what should it pay for that? Idzorek says when calculating the cost of moving to a better average fit, plan sponsors should be willing to pay a reasonable amount for an improvement. “If a plan sponsor can move to a QDIA that on average fits their plan population better by 15 equity percentage points, they should be willing to pay approximately 20 basis points for that type of improvement,” he says. “A cost greater than that would not justify a move.” Idzorek notes that there are a number of other benefits to managed accounts that aren’t considered specifically by this analysis, such as savings rate advice.
The same comparison of recommended equity allocation can also be used to evaluate a hybrid QDIA vehicle—one for which a target-date fund (TDF) is used for the younger demographic then participants would move to a managed account at a certain age. Idzorek says, “There’s not necessarily a right or wrong way to determine at what age the change to a managed account would occur, but if everyone younger than 35 is shown to be well-served by the glide path of the TDF and there is more dispersion above that age, then 35 would be the age for the transition to managed accounts.” He adds that the demographics of different plans could result in a different age. The key is that this new framework allows a plan sponsor to evaluate the options that are available to them, the costs, and given the plan’s unique demographics make a defendable, data-driven decision.
The comparison may also be used in more complicated scenarios. For example, Idzorek explains that if a plan sponsor offers a defined benefit (DB) plan, is considering freezing the DB plan, and changing their DC match, they may be wondering if their current target-date fund family is still appropriate for their plan given the potential changes. “Plan sponsors can incorporate information such as this to come up with specific equity recommendations for individuals under a variety of potential scenarios and use that information to inform their decision making,” he says.
“We have established a framework for quantifying the cost/benefit of a better-fit QDIA. As a result, plan sponsors can stop guessing and use participant demographic data to inform which … QDIA option … fits the plan best; the participant age or other factors that should be used to structure a hybrid QDIA; which glide path fits the plan best; and, whether a custom target-date glide path is necessary,” the paper says.
It notes that there is no agreed-upon definition of “best” when determining fit. The ideal implied glide paths for different plans can and should differ significantly. For some plans, a conservative glide path will be most appropriate, while for others an aggressive glide path will be most appropriate.
“We believe the techniques described in this paper will empower plan sponsors and their advisers to stop guessing and use an easy-to-implement, yet rigorous QDIA selection process,” the paper says. Idzorek adds, “The world is moving toward big data, and we haven’t been able to use big data in useful way until now. This new paradigm will enable plan sponsors to stop guessing and to meet their fiduciary obligations in a much more robust and defendable manner.”You Might Also Like:
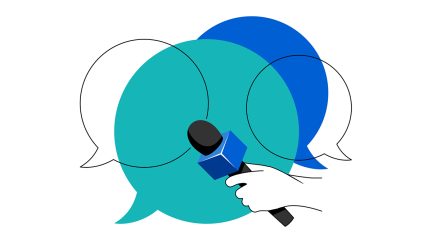
Stout Discusses Draw of National Role at Prime Capital Financial
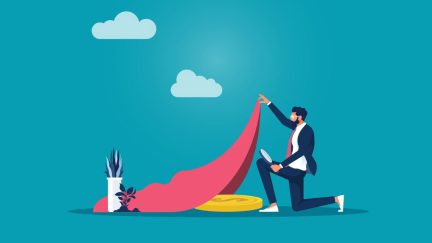
Implicitly Wrong: Hidden Fees in Retirement Income Solutions
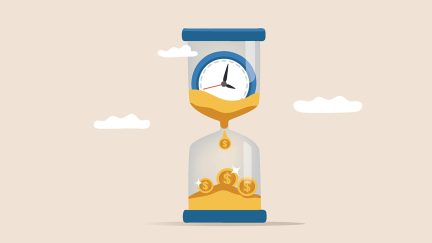